Compression-Based Methods for Statistical Analysis and Prediction of Time Series

Time series data, ubiquitous in various scientific and engineering disciplines, captures the dynamic evolution of a phenomenon over time. Understanding and predicting such data is crucial for decision-making, anomaly detection, and future forecasting. Compression-based methods have emerged as powerful tools for analyzing and predicting time series by leveraging the inherent redundancy and patterns within the data.
Compression algorithms, such as Huffman coding and Lempel-Ziv-Welch (LZW),identify and represent redundant patterns in data using shorter codes. The resulting compressed representation captures the essence of the data while reducing its size.
For time series data, the compression ratio provides insights into its predictability, with higher compression ratios indicating greater predictability. Compression techniques can also identify anomalies or abrupt changes in the data by detecting deviations from the compressed representation.
4.9 out of 5
Language | : | English |
File size | : | 3632 KB |
Screen Reader | : | Supported |
Print length | : | 153 pages |
Paperback | : | 102 pages |
Item Weight | : | 7.4 ounces |
Dimensions | : | 6 x 0.26 x 9 inches |
X-Ray for textbooks | : | Enabled |
Entropy, a measure of randomness or uncertainty in a system, plays a vital role in understanding time series data. Low entropy indicates that the data is predictable, while high entropy suggests randomness. Compression-based methods can estimate the entropy of a time series by computing the average length of the compressed representation.
This entropy estimation can be used to distinguish between different time series models, such as autoregressive integrated moving average (ARIMA) and seasonal ARIMA (SARIMA) models, and to identify suitable forecasting techniques.
Compression techniques can also be used for dimensionality reduction, particularly for high-dimensional time series data. By identifying the most important features or patterns in the data, compression algorithms can reduce the dimensionality while preserving the essential characteristics.
This dimensionality reduction enables more efficient and accurate statistical analysis and prediction, as it focuses on the most relevant variables.
In addition to analyzing time series data, compression-based methods can also be leveraged for predictive modeling. By identifying patterns and relationships within the compressed representation, machine learning algorithms can build predictive models that capture the underlying dynamics of the time series.
These models can then be used to forecast future values, identify trends, and make informed decisions.
Recent advancements in artificial intelligence (AI) and machine learning have spurred the development of sophisticated compression-based methods for time series analysis and prediction. Deep learning algorithms, such as convolutional neural networks (CNNs) and recurrent neural networks (RNNs),can exploit the hierarchical and sequential nature of time series data, respectively.
These algorithms can learn complex patterns and relationships within the data, enabling more accurate and robust prediction.
The choice of compression-based method for time series analysis and prediction depends on the specific characteristics of the data. Huffman coding is suitable for data with relatively short, repeating patterns, while LZW is more effective for data with longer, complex patterns.
For time series data with seasonal or cyclical patterns, SARIMA models combined with compression techniques offer improved prediction accuracy.
Compression-based methods have found wide-ranging applications in various domains, including:
- Financial time series forecasting
- Weather and climate prediction
- Medical signal analysis
- Industrial process monitoring
- Network traffic analysis
These methods provide valuable insights into the behavior of time series data, enabling better decision-making and more accurate predictions in critical areas.
Compression-based methods offer a powerful approach to analyze and predict time series data. By leveraging the inherent redundancy and patterns within the data, these techniques provide insights into its predictability, identify anomalies, and facilitate accurate forecasting.
As AI and machine learning continue to advance, compression-based methods will play an increasingly significant role in extracting valuable information and making informed decisions from time series data.
4.9 out of 5
Language | : | English |
File size | : | 3632 KB |
Screen Reader | : | Supported |
Print length | : | 153 pages |
Paperback | : | 102 pages |
Item Weight | : | 7.4 ounces |
Dimensions | : | 6 x 0.26 x 9 inches |
X-Ray for textbooks | : | Enabled |
Do you want to contribute by writing guest posts on this blog?
Please contact us and send us a resume of previous articles that you have written.
Novel
Chapter
Reader
Paperback
Magazine
Newspaper
Sentence
Shelf
Glossary
Preface
Synopsis
Footnote
Scroll
Codex
Tome
Narrative
Autobiography
Encyclopedia
Dictionary
Thesaurus
Narrator
Resolution
Librarian
Catalog
Borrowing
Periodicals
Research
Scholarly
Lending
Reserve
Academic
Journals
Reading Room
Interlibrary
Literacy
Thesis
Dissertation
Book Club
Theory
Textbooks
Gretchen Schultz
Ardenay Garner
Angela Jones
Julie Roy Jeffrey
Ted Efthymiadis
Alexis G Roldan
Katie Fforde
Donna Soto Morettini
Angie Herbertson
Caitlin Ring Carlson
Stephen J Cimbala
Niki Jilvontae
Roger Maxim
Margaret Thomson
Kelly Jones
Betsy Schwarm
Jeff Mays
J M Del Hagen
Michael L Wehmeyer
Brian Bilston
Light bulbAdvertise smarter! Our strategic ad space ensures maximum exposure. Reserve your spot today!
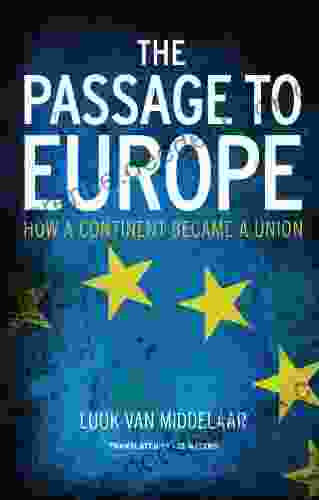

- Robert HeinleinFollow ·4.4k
- Rex HayesFollow ·16.2k
- Raymond ParkerFollow ·7.4k
- Gil TurnerFollow ·8.1k
- Rudyard KiplingFollow ·16.5k
- Eugene ScottFollow ·3.4k
- Billy FosterFollow ·9.4k
- Matthew WardFollow ·11.4k
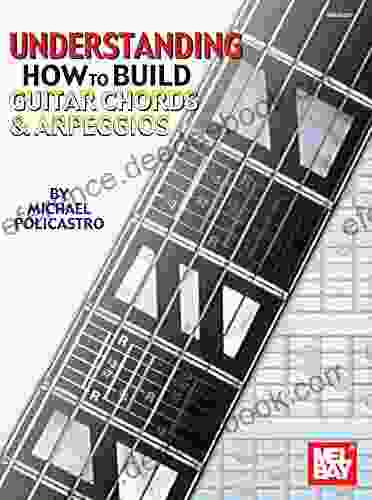

Understanding How to Build Guitar Chords and Arpeggios: A...
Mastering guitar chords and arpeggios...
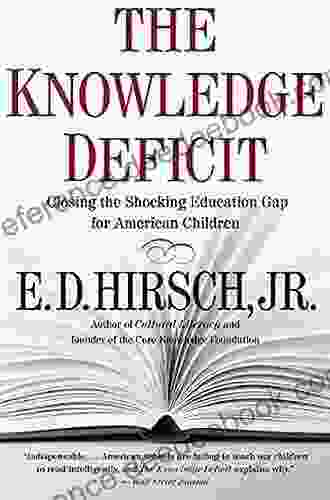

Closing the Shocking Education Gap for American Children:...
Education is the foundation...
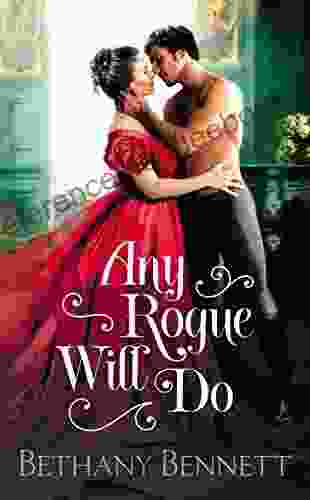

Any Rogue Will Do: A Captivating Adventure in the...
Step into the...
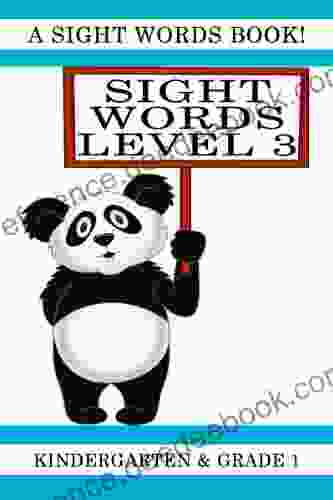

Mastering Sight Words Level 1: A Comprehensive Guide for...
In the realm...
4.9 out of 5
Language | : | English |
File size | : | 3632 KB |
Screen Reader | : | Supported |
Print length | : | 153 pages |
Paperback | : | 102 pages |
Item Weight | : | 7.4 ounces |
Dimensions | : | 6 x 0.26 x 9 inches |
X-Ray for textbooks | : | Enabled |