Big Data Machine Learning: A Comprehensive Exploration of Applications and Use Cases

4.8 out of 5
Language | : | English |
File size | : | 8884 KB |
Text-to-Speech | : | Enabled |
Screen Reader | : | Supported |
Enhanced typesetting | : | Enabled |
Print length | : | 118 pages |
In the era of digital transformation, businesses are sitting on a goldmine of data. This data, known as big data, holds immense potential for organizations to gain valuable insights, improve decision-making, and drive innovation. However, harnessing the power of big data requires sophisticated tools and techniques, and that's where big data machine learning comes into play.
Machine learning (ML) is a subset of artificial intelligence (AI) that allows computers to learn from data without explicit programming. When combined with big data, ML algorithms can uncover hidden patterns, identify trends, and make predictions from vast and complex datasets. This combination of big data and ML has revolutionized various industries, enabling businesses to achieve unprecedented levels of efficiency, accuracy, and personalization.
Applications of Big Data Machine Learning
The applications of big data machine learning span across a wide range of industries and domains. Here are some key areas where big data ML is making a significant impact:
Healthcare
* Disease Diagnosis and Prediction: ML algorithms analyze patient data, medical history, and genetic information to identify risk factors and predict the likelihood of developing specific diseases. This knowledge aids healthcare professionals in making informed decisions regarding preventive care and treatment plans. * Personalized Treatment: Big data ML enables tailored treatments by considering individual patient profiles, including their medical history, lifestyle, and genetic makeup. This approach ensures that patients receive the most appropriate and effective care. * Drug Discovery and Development: ML algorithms play a crucial role in identifying new drugs and optimizing drug development processes. By analyzing vast chemical databases and clinical trial data, ML models can predict drug properties, side effects, and efficacy.
Finance
* Fraud Detection: ML algorithms are used to detect fraudulent transactions in real-time by analyzing historical data on spending patterns, account behavior, and other relevant factors. This helps financial institutions prevent fraud and protect customers' financial security. * Credit Scoring and Risk Assessment: Big data ML models assess the creditworthiness of loan applicants by considering a wide range of data sources, including credit history, income, and debt-to-income ratio. This helps financial institutions make informed lending decisions and manage risk. * Investment Analysis: ML algorithms analyze market data, company financials, and news articles to identify investment opportunities and predict market trends. This aids investment professionals in making informed decisions for portfolio management.
Retail and E-commerce
* Personalized Recommendations: ML algorithms analyze customer purchase history, preferences, and demographics to make personalized product recommendations. This enhances the shopping experience and increases customer satisfaction. * Demand Forecasting: Big data ML models predict future demand for products based on historical sales data, seasonality, and other relevant factors. This helps retailers optimize inventory levels and avoid stockouts. * Sentiment Analysis: ML algorithms analyze customer reviews, social media posts, and other online content to gauge customer sentiment towards products and services. This feedback enables businesses to improve their products and services accordingly.
Manufacturing
* Predictive Maintenance: ML algorithms analyze sensor data from machines to predict potential failures and maintenance needs. This helps manufacturers proactively avoid unplanned downtime and optimize maintenance schedules. * Quality Control: ML algorithms analyze product images, sensor data, and other quality metrics to identify defects and ensure product quality. This reduces the need for manual inspections and improves operational efficiency. * Optimization of Production Processes: Big data ML models optimize production processes by analyzing data on machine performance, raw material consumption, and other factors. This enables manufacturers to reduce waste, increase productivity, and improve overall efficiency.
Real-World Use Cases of Big Data Machine Learning
To better understand the practical applications of big data machine learning, let's explore some real-world use cases:
* Netflix's Recommendation Engine: Netflix uses big data ML to analyze viewing history, ratings, and demographic data to make personalized movie and TV show recommendations to its users. This technology has significantly improved user engagement and customer satisfaction. * Amazon's Fraud Detection System: Amazon employs ML algorithms to detect fraudulent transactions in real-time based on data from purchase history, device information, and IP addresses. This system has helped Amazon reduce fraud by millions of dollars annually. * Walmart's Predictive Maintenance: Walmart uses ML algorithms to analyze sensor data from its forklifts to predict potential failures. This has reduced unplanned downtime and maintenance costs, saving the company millions of dollars.
Challenges and Opportunities of Big Data Machine Learning
While big data machine learning offers immense benefits, it also presents certain challenges and opportunities:
Challenges
* Data Quality and Complexity: Big data is often characterized by noise, missing values, and inconsistencies. Ensuring the quality and integrity of data is crucial for the success of ML models. * Computational Requirements: Training ML models on large datasets requires significant computational resources and can be time-consuming. Optimizing algorithms and leveraging cloud computing solutions can address this challenge. * Interpretability and Explainability: ML models can be complex and difficult to understand, making it challenging to interpret their results and explain their decisions. This can hinder trust and adoption of ML solutions.
Opportunities
* Continuous Learning and Improvement: Big data ML models can be continuously trained on new data, allowing them to adapt to changing conditions and improve their performance over time. * Collaboration and Innovation: Big data ML fosters collaboration between data scientists, engineers, and business leaders, driving innovation and the development of transformative solutions. * Ethical Considerations: The responsible and ethical use of big data ML is paramount. Addressing issues such as data privacy, bias, and accountability is essential to ensure the benefits of this technology are realized fairly and equitably.
Big data machine learning is a powerful tool that has the potential to revolutionize industries, transform decision-making, and create new opportunities. By leveraging vast datasets and sophisticated algorithms, businesses can gain valuable insights, improve their products and services, and optimize their operations. As the volume and complexity of data continue to grow, big data ML is poised to play an increasingly important role in shaping the future of business and society.
4.8 out of 5
Language | : | English |
File size | : | 8884 KB |
Text-to-Speech | : | Enabled |
Screen Reader | : | Supported |
Enhanced typesetting | : | Enabled |
Print length | : | 118 pages |
Do you want to contribute by writing guest posts on this blog?
Please contact us and send us a resume of previous articles that you have written.
Novel
Page
Text
Genre
Magazine
Sentence
Bookmark
Shelf
Glossary
Foreword
Preface
Synopsis
Footnote
Manuscript
Scroll
Codex
Bestseller
Library card
Autobiography
Dictionary
Character
Resolution
Librarian
Catalog
Archives
Study
Research
Lending
Reserve
Journals
Reading Room
Interlibrary
Literacy
Thesis
Dissertation
Storytelling
Awards
Reading List
Book Club
Textbooks
Natalie Del Favero
Justus D Doenecke
Anna Wilkinson
Lazaro Wolf
David N Feldman
Avi Katz
Justin Wainwright
Lisa E Scott
Amy Snow
Jerome Sitko
Matt Artz
Nyria Fey
Susan Penny
B B Blaque
Robert Levine
Elizabeth Drake
Tracey Mclennan
Gino Rubert
Domenico Piccolo
Sandy Walker
Light bulbAdvertise smarter! Our strategic ad space ensures maximum exposure. Reserve your spot today!
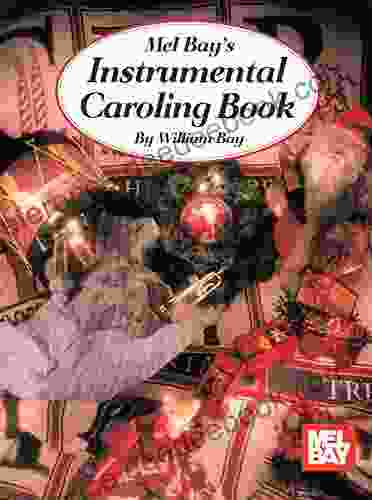

- Marc FosterFollow ·10.5k
- Fabian MitchellFollow ·15k
- Roger TurnerFollow ·4.4k
- Clark BellFollow ·17.7k
- Gage HayesFollow ·16.6k
- Ernest J. GainesFollow ·18.6k
- Terry BellFollow ·19.7k
- Elliott CarterFollow ·6.8k
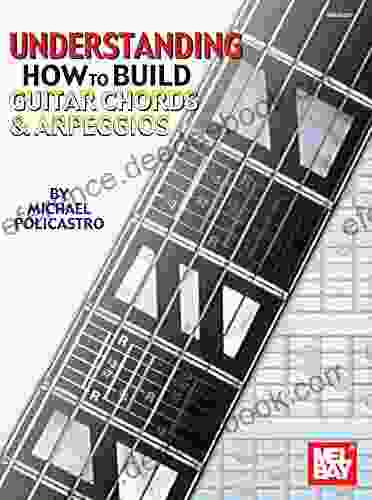

Understanding How to Build Guitar Chords and Arpeggios: A...
Mastering guitar chords and arpeggios...
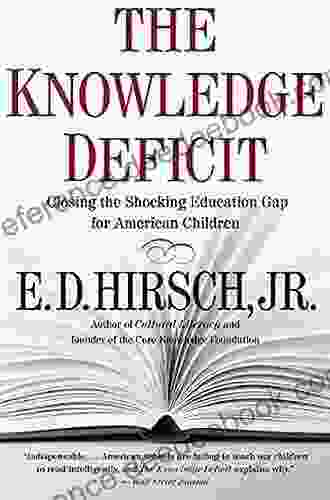

Closing the Shocking Education Gap for American Children:...
Education is the foundation...
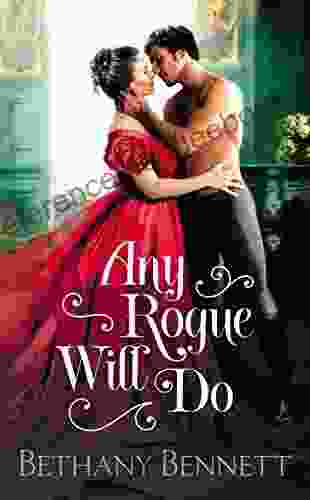

Any Rogue Will Do: A Captivating Adventure in the...
Step into the...
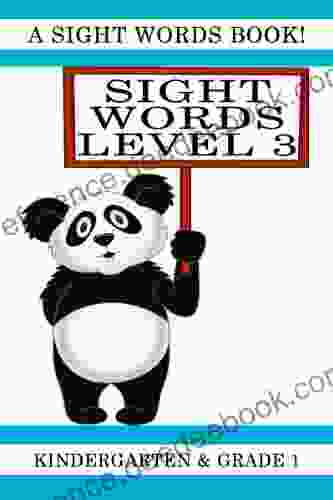

Mastering Sight Words Level 1: A Comprehensive Guide for...
In the realm...
4.8 out of 5
Language | : | English |
File size | : | 8884 KB |
Text-to-Speech | : | Enabled |
Screen Reader | : | Supported |
Enhanced typesetting | : | Enabled |
Print length | : | 118 pages |